Robotics and Artificial intelligence showcase 2019. (write-up)
Robotics and Artificial intelligence showcase was held in Manchester, 12th of March 2019.
Radio links
The Oil & gas technology centre had a really nice point cloud of oil rig, down to the minute detail (video below).
The centre provides an umbrella for a number of smaller companies, one of which is the drone operator Air control entech. Air control entech operates large drones,, having operations and drone pilots.
Clogworks manufactures drones (with distinct aesthetics), and CEO provided very interesting insight in radio link dependency. In particular, the main competition is from DJI with Lightbridge 2 radio link (5km range). Clogworks is happy with their new radio link 20km, 25Mbps (in co-operation with Sky-drone). The next step for Clogworks is further increasing the range and size of their drones (100kg payloads, e.g.).
SMC corporation from Tokyo has an industrially used radio link (one master, 6slaves, 250kbps, frequency hopping – for security reasons, 5ms delay) EX600-W. The links is used for robotic arms where due to the movement the data link cable gets broken often, or where the robotic arm needs a full 360degrees movement at the pedestal. SMC is the only vendor of wireless solutions for robotics in the UK.
Robotics and AI in nuclear (RAIN) is of potential interest for small cells community and they will have:
- a demo day on 26th March
- assurance on 12th April (NB: to provide more info here)
- game changers on 19th September (NB: to provide more info here)
Robotic arms
University of Liverpool has active co-operation with Kuka robots, and has won some awards from Kuka challenges.
ABB has reserves with wireless communication from the safety – risk point of view. ABB does not, as of 2019., manufacture moving robotic platforms.
Automata exhibited their Eva robotic arm for less than £5,000.
Computer vision
Manufacturer of European Mars rover, SCISYS are moving away from nVidia (clearly for radiation hardening reasons).
London based, Sensing feeling has a low power solution (Jetson TX2 –> Xavier, and Intel Atom + Movidius VPU platform – I assume Myriad X) for face and feelings recognition.
(There is a lingering but not confirmed assumption that FPGA solutions have less delay/power comparing with GPUs.)
MWC 2019. (write up)
Mobile world congress in 2019. was just a slight improvement against the last year MWC. The main theme changed from selfdriving onto robotics and computer vision. Both selfdriving and robotics are powered by AI (Artificial intelligence). And 5G was everywhere (and real 5G modems, not power point modems, as per Huawei).
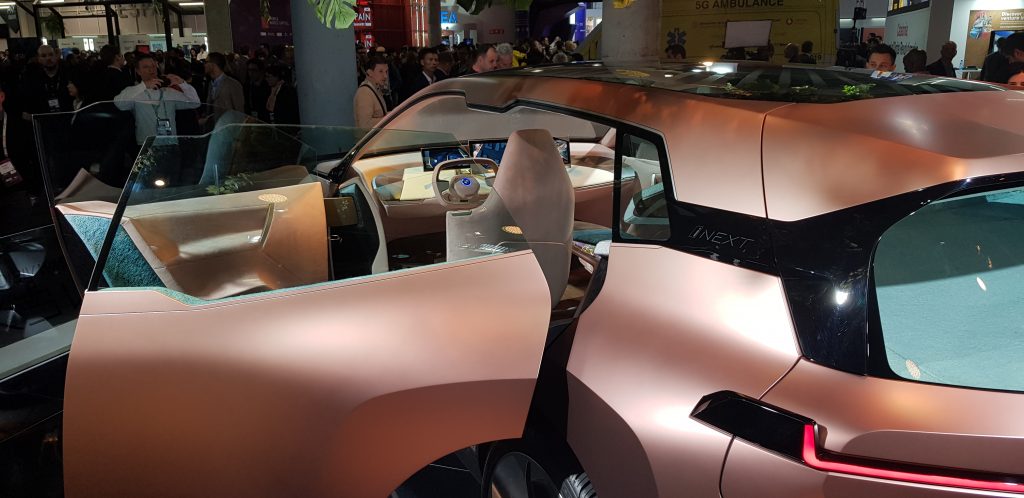
Software defined radio
FaceBook, together with many operators is trying to support open source community to develop ecosystem that could compete against Huawei, named Telecom infrastructure project (TIP). One part of the TIP is CrowdCell which was presented in FaceBook’s booth, and for the purpose of demo, integrated with Athonet nVidia GPUs server.
CrowdCell is an SDR platform running on Lime mycrosystems SDR platform. The main value Lime claims to bring are their RF chips (as opposed to an usual SDR solution which would use a combination of different chips, which might not satisfy commercial power/ dimensions requirement, Lime packaged all RF baseband processing into a single chip). Lime investors include DFJ Capital of Steve Jurvetson fame. The suspicion is that VC is targeting AI + SDR combination. Myriad RF and bladeRF are using Lime chip, in combination with Altera FPGA. (Altera can be replaced by Xilinx if needed.)
Benetel is another SDR company, member of TIP, which beside base stations is doing UEs based on SDR. (Note: I was told about Benetel by Euricom.)
Artificial intelligence
Huawei
Huawei AI chips Huawei AI data centre card (left) and other AI chips (right)
Huawei came out with full spectrum of AI chips (embedded) and cards (PCIe). (Note: For a brief moment I was there, it was almost an empty stand with more interest from Chinese onlookers than anyone else – presentation was in Chinese.)
The table below contains some comparison between Huawei AI and the rest.
(While Huawei effort is commendable, there’s a long way to catch up.)
Manufacturer | AI chip | TOPS | Power (Watt) |
Huawei | Ascend 310 (SoC) | 16TOPS Int8 | 8W |
Atlas 200 (?) | 16TOPS Int8 | 9.5W | |
Atlas 300 (PCIe) | 64TOPS Int8 | 75W | |
nVidia | Xavier (SoC) | 32TOPS (Int8?) | 10W |
T4 (PCIe) | 130TOPS Int8 260TOPS Int4 | 75W | |
Intel | EyeQ5 (SoC) | 24TOPS (Int8?) | 10W |
Xilinx | Zync (RFSoC) | ? | ? |
Virtex VU9P (FPGA PCIe) | 21TOPS Int8 | 75W | |
TPU (?) | 45TOPS Int8 | ? |
Xilinx
Xilinx has on its stand an impressive Zynq RFSoC solution (8×8 MIMO) and Virtex xDNN FPGA card.
Main job for FPGA card is image recognition, and to lesser extent speech processing. There’s a new versal architecture on 7nm coming end of the year.
(There’s something strange with Xilinx numbers. It’s not clear why FPGA cards are so successful.)
ORBL
Orbl.io is a small Estonian company providing 5x DNNs to determine face, age, gender, emotions and liveliness of a person. Orbl sales are in Bay area.
Computer vision
Israeli stand
Israeli stand is again bigger this year. It always seems to be the most innovative and commercially ready stand.
Ionterra presented a SW solution for object recognition, tailored for low power devices (Raspberry pie or ARM, e.g.) which would achieve high FPS (Frames per second) without using DNNs. The nature of the algorithm was not revealed, but it’s clear to see the need (e.g. emergency shutdown of a robot has to be controlled locally.)
Elsight had a highly networked solution. E.g. 4x radio links of 4x different operators from a single base station.
Miscellanea
A Supermicro product manager was asked about DRAM pricing. The answer was that Supermicro has no pricing power when ordering memory and pays what suppliers ask. (If any negotiation takes place, memory supplier brings dubious arguments of fab flooding or similar, to maintain DRAM price.)
This DRAM contract price not going down is interesting. Definitely, the reduction in price is what would be expected based on DRAM price cycles.
One of Small cell forum vice-chairs is now with BEC technologies.
In the new technologies hall 8, an interesting information on a start-up doing quantum computing with entangled photons, based on silicon photonics. PsiQuantum (hiring) is led by ex Bristol University and Imperial college professors Jeremy O’Brien and Terry Rudolph (Erwin Schroedinger’s grandson).
Term 1 – Robotics
ROS Essentials, Perception, and Control
Project: Search and sample return
Introduction to ROS
Packages and catkin workspaces
Biologically inspired robots
Write ROS nodes
Intro to kinematics
Forward and reverse kinematics
Denavit Hartenberg parameters for serial manipulators.
Project: Robotic arm: Pick and place
Human robot interaction & robot ethics
Perception overview
Introduction to 3D perception
Calibration, filtering and segmentation
- Intro to Calibration, Filtering, and Segmentation
- Sensor Calibration
- RGB Camera Model
- Calibration Pattern
- OpenCV Calibration
- Extrinsic Calibration
- RGBD Calibration in ROS
- Point Cloud Filtering
- Tabletop Segmentation Exercise
- Voxel Grid Downsampling
- Pass Through Filtering
- Segmentation in Perception
- RANSAC Overview
- RANSAC Plane Fitting
- Extracting Indices
- Outlier Removal FilterClustering and segmentation
Object recognition
Project: 3D perception
Soft robotics
Robot grasping
Introduction to controls
Quadrotor control using PID
- Introduction to a Positional Controller
- Quadrotor Kinematic and Dynamic Model 1
- Quadrotor Quiz
- Quadrotor Kinematic and Dynamic Model 2
- Cascade PID Control
- Lab Walkthrough
- Environment Setup
- Exploring the Sim
- Helpful Tools
- Completing PID Controller
- Hover Controller
- dynamic_reconfigure
- Attitude Controller
- Positional Controller
- Lab Summary
- PID Wrap Up
Swarm robotics
- Introduction
- READ : Research Papers
- Swarm Robots in Medicine
- Kilobots
- Search and Rescue
- Self-Assembly Swarm Robots
- WATCH: Concepts in Action
- Meet Sabine Hauert
- Exclusive: Sabine Hauert
- DO : Lab – Create a Swarm
Intro to neural networks
Deep neural networks
Convolutional neural networks
Fully convolutional networks
Lab: Semantic segmentation
Project: Follow me
Introduction to C++ for robotics
MWC 2018. (write up)
Those thinking 2018. MWC lacked sparkles, obviously didn’t see dazzling 3D Hypervsn(TM).
AI
All mobile processor manufacturers have added, next to graphical processors, Artificial intelligence (Neural networks) engine to their ARM processors.
- ARM added Mali
- MediaTek (in P60) added APU
- Qualcomm added Adreno
There was no information on Huawei, and Apple AI processors – but they doubtlessly exist.
Israeli stand
An amazing inference chip, working entirely on optical principle was proposed by CogniFiber. (Each pixel has its own fiber of, say 30um, out of say, 600x400px. Thresholds of neural network are probably implemented as amplifiers for undersea fibers – no slowing down of light. Claim is: about 1000x less power and a few orders of magnitude speed up.)
Israeli syte.ai has a mobile app which can take camera photo to identify clothes (brand) on the person one envies and finds the price and where to buy it.
Another company has a ‘self-driving’ system for the passenger – one gets all the information about shops, restaurants, hotels, etc… on the camera photo, while being a co-driver.
SDR/URLLC
Fraunhofer institute demonstrated shortened TTI implemented in USRP.
CellXica from UK developed their own SDR platform and base station. (Thanx Prashant for tapping my shoulder.)
Other
SoftBank robotics brought childlike robots Nao and Pepper which were popular with women.
Memory
Intel True VR(TM) needs 2TB per hour (6 pairs of camera capture of 360degrees.)
Cars
Audi A8 has about 50x ARM processors.
(Ridiculous) concept car on Mercedes stand.
Graphene
Graphene corner was in Hall 8 just as the last year, and the monetisation remains the problem. Among other applications:
- Transparent graphene film over window’s surface. (Maybe in future we will have each window as a TV?)
- All kind of sensors
- Thermal conductor (better than copper)
Braincom-project.eu has increased the number of brain implanted electrodes from few hundred, to, using graphene, few tens of thousands of electrodes.
Russian stand
On quite modest Russian stand one could have seen Sailfish OS – alternative to iOS, Android and Windows – used by government. (It’s a Linux flavour.)
Term 3 – Selfdriving
Path planning, concentrations and systems
Path planning
- Search:
Discrete path planning and solving algorithms (A*). - Prediction:
Sensor fusion used to predict other objects behaviour. - Trajectory generation in C++:
Project: Path planning
Drive a car down a highway with other cars using one’s own path planner.
Advanced deep learning
- Fully connected convolutional networks
- Scene understanding
- Inference performance
- Project: Semantic segmentation
Autonomous vehicle architecture
- Introduction to ROS (Robot operating system):
Architectural overview of ROS framework and setting up the environment. - Packages & Catkin workspaces:
ROS workspaces structure, essential command line tools and software package management - Writing ROS nodes:
Python and C++. - Project: System integration project
Running the code on Carla, Udacity’s autonomous vehicle.
Inaugural class of Udacity Self-driving nanodegree graduation celebration with Sebastian Thrun.
Press release – Private ePC plugfest
Small Cell Forum and ITRI take leap forward in private networks
Technical support for the event was provided by SoftBank, ITRI and Magnum, a.i.
Vehicle detection
MWC 2017. (write up)
- Meeting with Small ePC vendors, possible participants of Small cell forum Private ePC plugfest (in the role of Small cell forum Interoperability chair).
- Status of DNN implementations
(Note: companies maybe didn’t bring the best they have, otherwise, it’s bad):
NXP had brought a range of radar chips, Lidar and ultrasonic chips. Sensor fusion is done in “blue box” powered by 4x ARM cores. (Implementation doesn’t seem perfect: Lidar doesn’t recognise all the people, and point cloud has artefacts, while camera recognises people where there are none.)
Vodafone had Huawei‘s demonstrator for 5G (and possibly autonomous driving) running car simulation in PlayStation 4 (it seems Huawei doesn’t like x86 technology – I wouldn’t be surprised if Huawei and Sony announce partnership – for microprocessor/GPU/sensors, as Huawei is cut-off from top US tech.) Car simulation was not good/ convincing – if autonomous driving algorithms were used (and simulation had road-works/ cyclists/ pedestrians…).
I observed a simulation similar to Huawei’s on Orange stand.
HP Enterprise seemed to have car simulator similar to what we used, based on Unity engine. (Again, the driving skills of algorithm/ human were suboptimal.) HP Enterprise doesn’t seem to have a device for SMEs (a mistake, I think – they should be called HP for big Enterprise)
Qualcomm had on their stand SnapDragon with DNN support through Adreno (GPU that Qcomm bought from AMD some time ago) manned by a Chinese person. A lonely stand, except for one more Chinese visitor – so the conversation was in Chinese. Ni hao. Not impressive (particularly in the light what other companies think about DNN on SnapDragon).
SQREAM (from Israel) had SQL developed for GPUs. Very good solution. They mentioned (and the similar strategy will be used by all other companies) that they can swap AMD for Nvidia, but will never maintain two separate product lines (for AMD and Nvidia GPUs). Claim was that AMD improved a lot, but they stick with Nvidia. (And this is likely how AMD/ Nvidia “war” will end-up.)
From Israeli stand, I got some indications how quick DNNs in mobile deployment, generally are.
- SDR
NI (National instruments) had a very nice 5G setup.
LimeSDR, Octasic, …
- I was introduced to James Tagg, the director of Penrose Institute in La Jolla (Sir Roger Penrose is the best physicist in the world, in my opinion). James is involved with two fascinating projects:
To predict when NN can fail (not clear if this is a computable problem) – Anyone who trained NN knows it can fail unpredictably – solution to this problem will help DNN deployments tremendously.
To prove professor Penrose’s objective reality of quantum physics, by gravity induced collapse of quantum wave functions through an experiment.
How unlikely to meet such a person at an event like MWC. (Thank you James to introducing me to James, and thank you James for being so kind and having time for the chat.)
- Other
I spoke with Spanish satellite defence contractor, on the combination of Phased array antennae with Luneburg sphere.
I met with Jean-Francois Lacasse of Cavium (who I first met through Small cell forum plugfest in Paris 2014) and had a nice chat – how things are turning great for multi-core ARM Cavium server. (He’s involved with LimeSDR and Ubuntu, and will chair Open Cellular)
I visited 3D mapping companies (possibly to integrate their maps in Unity game engine). (LuxCarta ladies were especially nice, and gifted me with all sorts of gadgets.)In general, it seems more hype than money. Time for change…
( Hall 1: people who bought their way in.
Hall 2: companies that GSMA seriously believe contribute to industry and are making money
Hall 3: old companies, old money
Hall 4: conference rooms
Hall 5-7: small companies, and pavillions per country (Irish one is always the one with the best beer))
Advanced lane finding
And when algorithms fail, below: